In our ever-evolving world, the integration of artificial intelligence (AI) into our daily lives continues to shape our future. One fascinating area of AI research is Long-Term Action Anticipation, a concept that has the potential to enhance human-robot collaboration for better proactive robot behavior. Imagine a world where robots not only understand what we’re doing now but can also predict what we’re going to do next. Picture a future where your AI assistant knows your intentions before you even voice them. This is the promise of Long-Term Action Anticipation (LTA).
The Need for Long-Term Action Anticipation
In our everyday lives, whether we’re cooking dinner or working in a team, anticipating future actions is essential. Humans have an innate ability to foresee the next steps in a complex task. We can understand that when someone picks up a knife, they’re likely going to use it to cut something. This predictive power enables us to collaborate seamlessly with others.
However, transferring this intuitive skill to robots has proven to be a complex challenge. The future is inherently uncertain, and people often have unique ways of doing things. This is where LTA comes in. By developing AI systems that can read and interpret human intentions, we open up new possibilities for improved human-robot collaboration and personalization.
Enhancing Human-Robot Collaboration
One of the most exciting applications of LTA is in the field of human-robot collaboration. Imagine working alongside a robot that not only performs tasks efficiently but also understands your intentions and can adapt accordingly. This level of collaboration has the potential to revolutionize industries like manufacturing, healthcare, and even everyday household chores.
For instance, if you’re cooking with a robot assistant, it can anticipate your next steps based on your overall goal, such as making a salad. This means it can prepare the ingredients you need before you even ask, making the entire process smoother and more efficient.
Personalization Redefined
Personalization is another frontier that our research delves into. LTA offers the potential to create AI systems that truly understand individual needs and preferences. These systems can learn from past interactions and anticipate user actions, providing a level of personalization that goes beyond conventional AI assistants. From tailored recommendations to anticipatory responses, the possibilities are limitless.
Our Contribution
The PERSEO project has made interesting strikes in the long-term human action anticipation from videos. In our work, titled Intention-Conditioned Long-Term Human Egocentric Action Forecasting, we present a methodology that aims to narrow down the variability of future actions based on inferred human intentions from past observations. We introduce two critical components: a Hierarchical Multitask Multi-Layer Perceptrons (MLP) Mixer (H3M) for action classification and intention extraction, and an Intention-Conditioned Variational Autoencoder (I-CVAE) for predicting future actions based on intention and past actions. These components work together to create a framework that demonstrates the effectiveness of LTA.
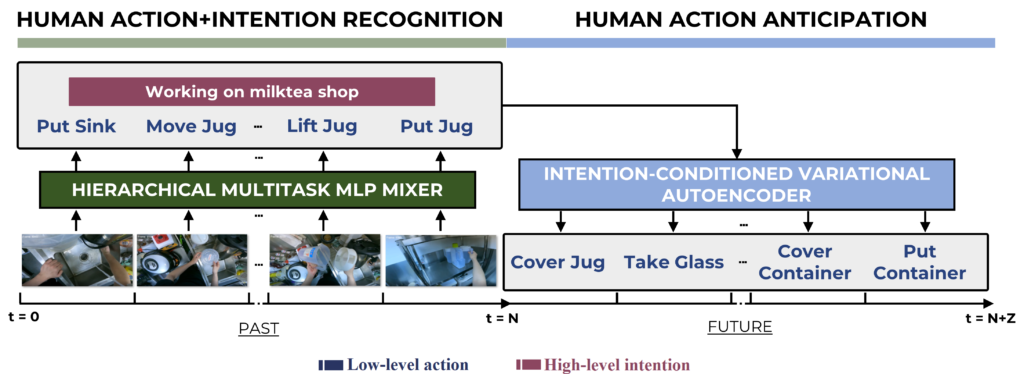
We tackle this LTA task in the largest egocentric dataset available, Ego4D, and showcase that our approach outperforms the state-of-the-art in the Ego4D LTA task. Our work ranked first in both CVPR@2022 and ECCV@2022 Ego4D LTA Challenge from MetaAI, which demonstrates the good direction of research of the PERSEO Project.
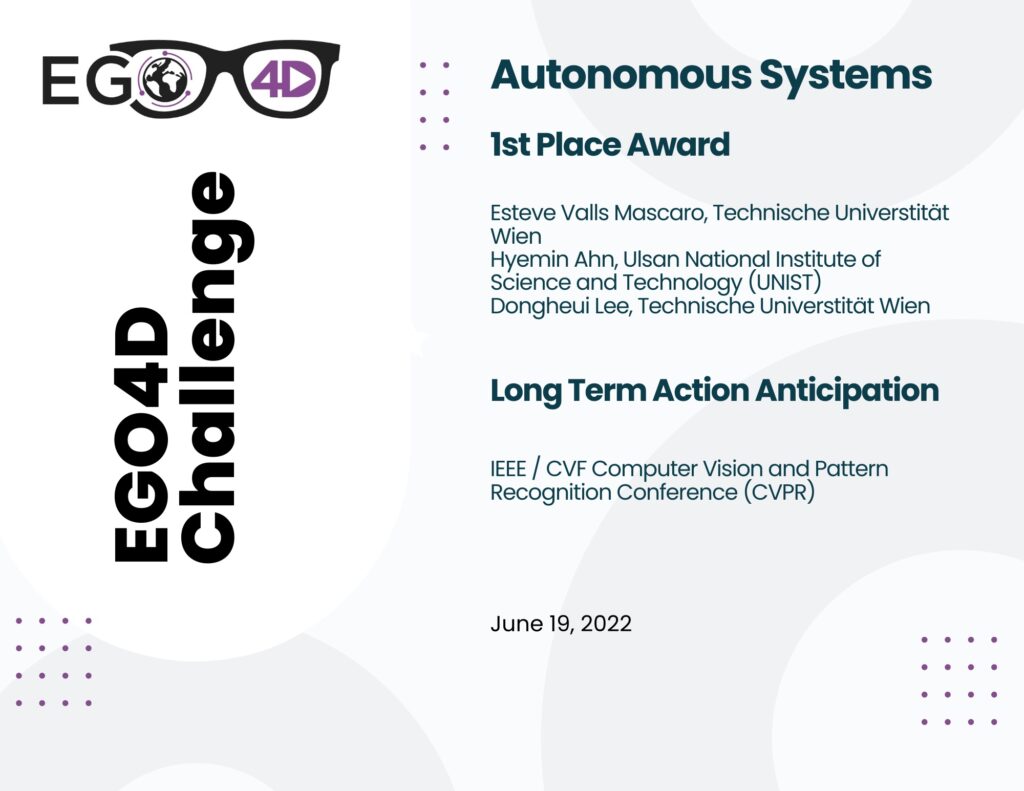

The Future of AI
As we continue to advance in AI research, Long-Term Action Anticipation is a significant stepping stone toward a more intuitive integration of robotics into our lives. The journey to achieving this level of AI sophistication is ongoing, but the potential benefits are undeniable. The future is bright, and it’s filled with AI and robots that not only understand what we’re doing but also anticipate what we’re going to do next. Our PERSEO project will continue its research to make our interaction with robots easier, more efficient, and more personalized than ever before.